Meg analysis pipeline
Reproducible MEG Analysis
MEG data collection and analysis requires a lot of interacting components (Stimulus delivery, logfiles, projector onset triggers, analog and digital triggering, electrophysiology measures, MRI coregistration...) that can lead to heterogeneous data and data quality. This makes pipeline analysis particularly difficult due to the variability in inputs. Additionally, with the multitude of techniques and metrics to evaluate MEG data (dipole/MNE/dSPM/beamformer/DICS beamformer/parametric stats/non-parametric stats ....) projects can have evolving analysis protocols. Knowing what preprocessing was performed on what datasets can often come down to an excel spreadsheet or text document. Since these projects can sometimes last multiple years, it is important to know what was done to produce a specific result and how to apply these analysis techniques to new incoming data.
Containerized Computing Environment
#It can be easy to build a container from a current conda environment file conda env export >> environment.yml #It may be necessary to remove some components such as VTK
#Create a singularity def file (textfile) from below Bootstrap: docker From: continuumio/miniconda3 %files environment.yml %post /opt/conda/bin/conda env update --file environment.yml --name base %runscript exec "$@"
#Build container using singularity sudo singularity build conda_env.sif def_file.def
Nipype
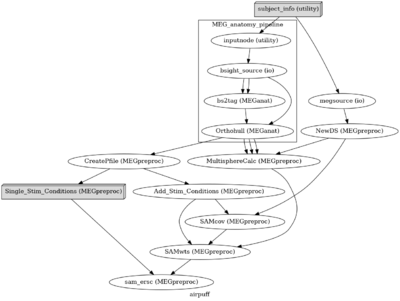